How Artificial Intelligence is Reshaping Drug Discovery
How can artificial intelligence enhance drug discovery, reduce cost, and bring new drugs to market faster? In this article we explore how AI is reshaping drug discovery as we know it.
Here’s a harsh reality check: 96% of potentially life-saving drugs never make it to market. Excessive costs, ranging from $1.3 billion to $2.8 billion, and steep regulatory burdens make it difficult for novel therapies to penetrate the market.
On average, it takes 12 to 15 years of testing for a drug to move from the laboratory, through clinical trials, and finally to FDA approval.
The bottom line? Only one out of every 5,000 drugs screened make it to market.
Yikes.
This begs the question: How can artificial intelligence (AI) powered technology enhance drug discovery, reduce cost, and bring new drugs to market faster?
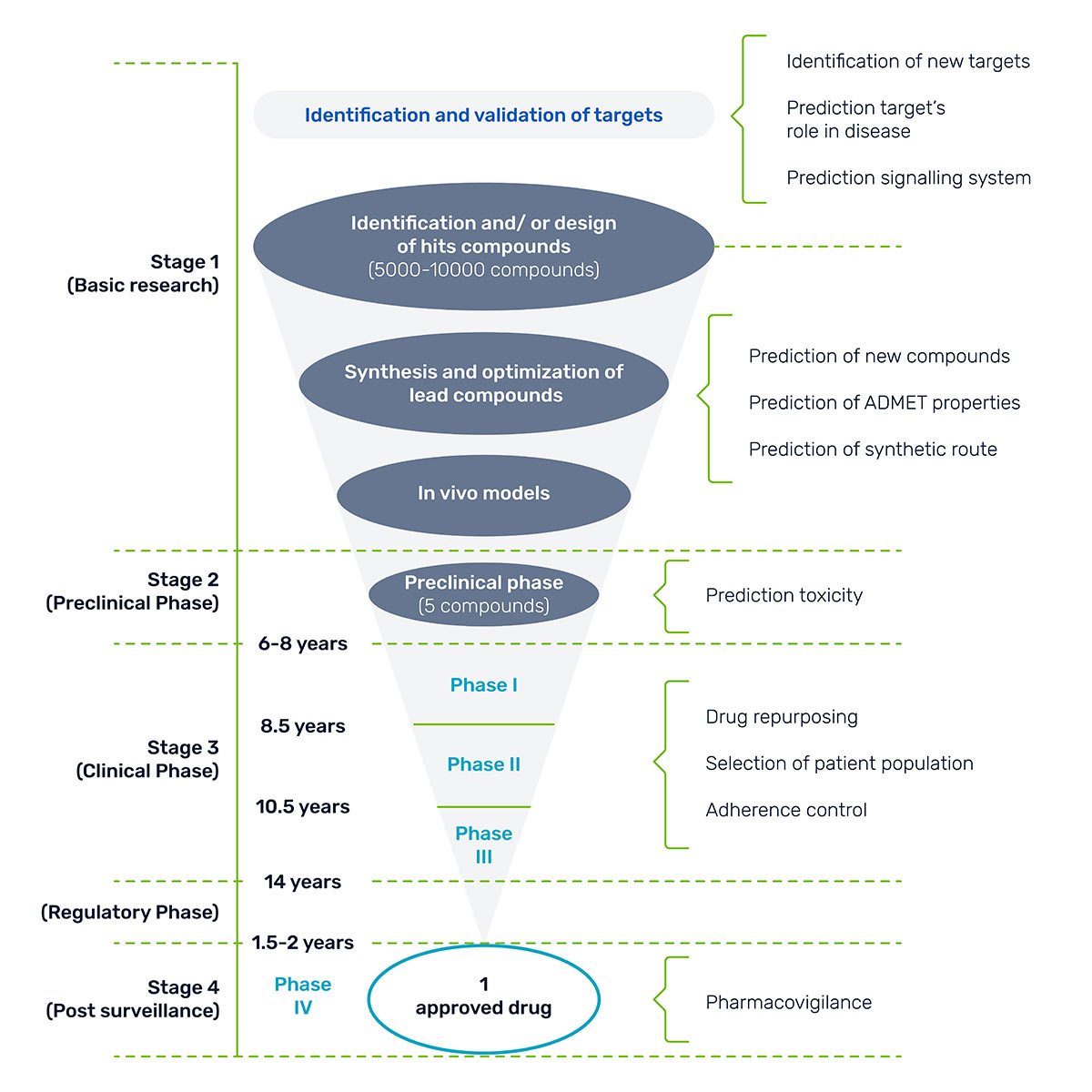
Figure 1: Timeline for drug approval.
Is the COVID-19 Model the Future of Drug Development?
Under normal circumstances, the COVID-19 vaccine would have taken longer than a decade to move through the discovery, clinical trials, and the approval process. However, desperate times call for desperate measures, which is why the Federal Drug Administration (FDA) launched a new program known as the Coronavirus Treatment Acceleration Program (CTAP).
The purpose of this program was to “help move new medical products to patients as soon as possible, while at the same time determine whether they are effective and if the benefits outweigh the risks.” By merging traditional methods of drug discovery with AI, drug screening became more effective via the CTAP, thereby resulting in a faster discovery and approval process.
And it worked.
Last year BenevolentAI, a London-based firm, successfully identified baricitinib – a drug created to help rheumatoid arthritis patients – as a strong candidate for COVID-19 treatment. Through AI-powered knowledge graphs, BenevolentAI identified anti-inflammatory and anti-viral compounds in baricitinib in less than 48 hours.
This proved to be a breakthrough, as severe coronavirus is associated with dysregulated inflammation (meaning immune response goes into overdrive, and the virus can spread more quickly through the body). As of now, baricitinib is FDA-approved.
The Rise of AI and Machine Learning in Drug Development
While researching for this article, we uncovered 235 organizations currently leveraging AI and machine learning (ML) for pharmaceutical research and development (R&D). With major industry players such as Exscientia AI, Pfizer, and AstraZeneca leading the way, the adoption of AI and ML for drug development will continue to increase.
In 2015, investors funneled $500 million into AI-powered pharmaceutical R&D. In 2021, the investment amount increased to more than $4.4 billion — resulting in a CAGR of 43.6%. And growth is not expected to slow down anytime soon. Instead, researchers predict the market value will grow to more than $20 billion during the next five years.
The increase of AI-powered R&D adoption corresponds with the growth of investments, as well as the number of startups entering the market.
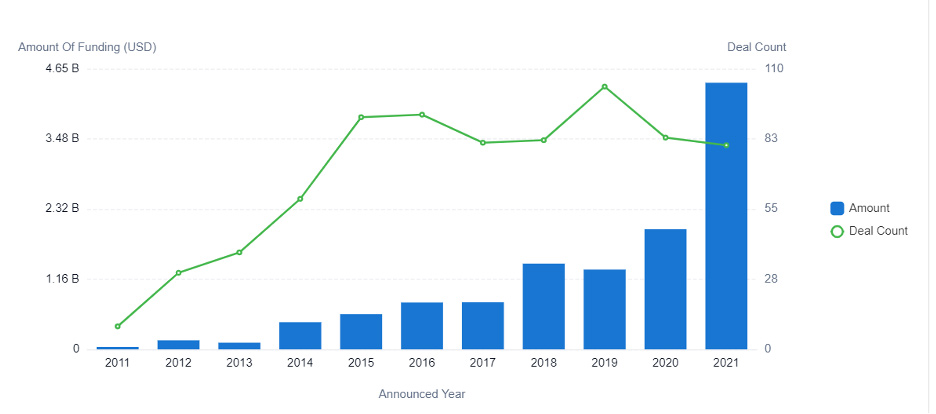
Figure 2: This graph displays the investments in AI for drug development between 2011 to 2021.
How AI is Reshaping Drug Development
A drug discovery program initiates when there is a specific disease or illness that does not have sufficient medical products available. In many cases, researchers are not searching for a novel structure, but instead are adapting the data from 20,000 existing drug products that are already approved for marketing. These drugs have a wealth of literature, clinical data, and IP that can be utilized to discover novel therapies; the difficulty is applying the data from existing drugs to combat new sicknesses.
But there’s one massive mountain researchers have to climb: public data is largely unstructured and fundamentally different. This means not only are these data sources difficult to analyze (in the traditional sense), they’re also disparate.
In order for researchers to extract meaningful insights (before it’s time to retire), these disparate datasets need to be connected, organized, and validated for accuracy.
That’s where AI comes in — branches such as natural language processing (NLP) can analyze immense amounts of natural language data, understand the contextual nuances, and link drugs, targets, and expected therapeutic outcomes in a matter of minutes.
Connected Innovation Intelligence (CII) platforms like PatSnap’s Synapse link literature, patents, and sequence data to help researchers with their preclinical screening process. Plus, researchers can create knowledge graphs, thereby reducing the time it takes to find proper drug candidate.
As BenevolentAI demonstrated, knowledge graphs are gamechangers. They can help researchers make connections between drugs, targets, and disease that would normally be deemed unrelated. Once these relationships are discovered, researchers can act upon these insights by making predictions, modelling novel pathways, and testing their findings.
To learn more about PatSnap’s AI-powered Connected Innovation Intelligence platform and how it can help your company accelerate drug discovery, download our Definitive Guide to Connected Innovation Intelligence.
Author Bio
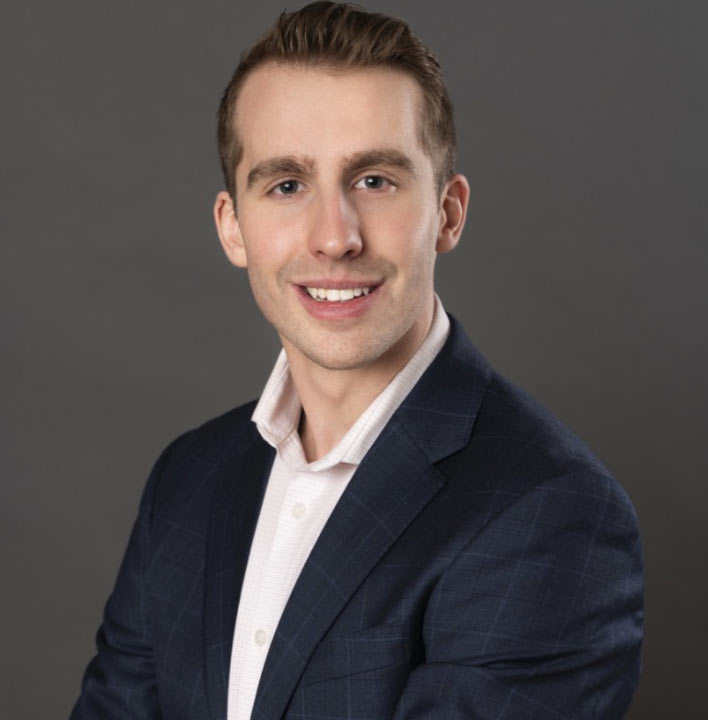
Christian Agueci is a Project Manager on the client growth team at PatSnap. He graduated from Laurier University with an undergrad in biochemistry and a Master of Business Administration. During Covid, Christian took up a few hobbies including gardening and running. He plans to run his first marathon in the spring of 2022.